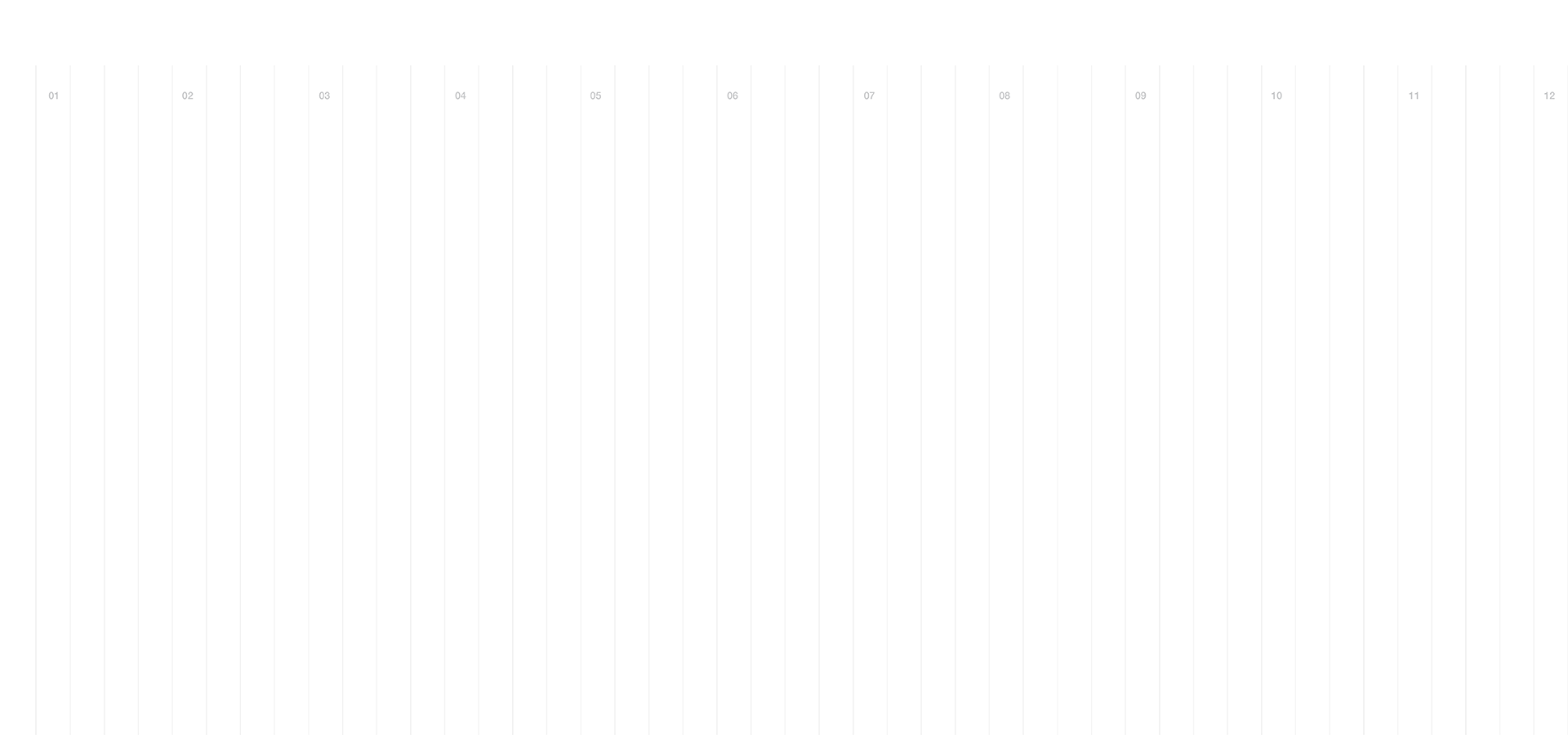
Practical AI
In today's business landscape, practical AI solutions offer tangible benefits without the worry of apocalyptic consequences. AI has real, measurable returns that you can access today. And we know how to make AI work for you.
Practical AI
You must be able to measure your success or no one in your company will see the impact you’ve made. DBMT success metrics reveal AI implementation value for each team and business unit. The key is accurately and demonstratively proving this value
​
Related DBMT Products and Services:
PRACTICAL AI: STRAIGHT TALK
​
Let's cut through the noise. In today's business landscape, practical AI solutions offer tangible benefits without the worry of apocalyptic consequences. AI has real, measurable returns that you can access today. And we know how to make AI work for you. The returns on AI investment is three-fold:
-
Faster delivery of content and services
-
SME empowerment and efficiency
-
Institutional knowledge retention regardless of personnel changes
​
​
DBMT’s unique approach to standing up AI/LLM capabilities in your organization include:
-
Knowledge Domains that deliver Accuracy and Value: Instead of just focusing on the best model or workflow, our key differentiator lies in our hyper-focus on developing robust Knowledge Domains through DBMT’s exceptional KnowS methodology, delivering perhaps the most important aspects of an AI solution – Information Accuracy and Relevance.
-
Impact and Quality Measurement that ensures continual improvement and achievement of desired outcomes: DBMT provides the tools and processes you need to achieve value and shape the success of your AI implementations in four essential areas - Efficiency, SME Empowerment, Accuracy, and Relevance.
-
Optimized Set of AI Tools and Solutions designed to deliver business-grade performance and accuracy. DBMT provides you with a curated set of models, workflows, platforms and interfaces that help you stand up AI/LLM capabilities within your organization and prioritize tangible results, ensuring you see a substantial return on your AI investments.
The Necessity of Knowledge Domains: In the landscape of AI and LLM solutions, the performance of applications is often constrained by the quality and structuring of input data. Despite continual improvements in LLM solutions, meeting AI high expectations relies on establishing Knowledge Domains rather than Company Knowledge aggregation alone. Pre-Optimized Inputs, structured as Knowledge Domains, ensure the required accuracy, relevance, and performance, irrespective of the advancements in LLM/AI technology. To accomplish this, DBMT establishes an automated workflow for the curation and processing of Company Knowledge. This includes:
-
Self-Referential Conflict Resolution:
-
Identification and resolution of contradictory information within the Knowledge Domain, ensuring internal consistency and reliability.
-
-
Knowledge Gap Resolution:
-
Use of DBMT Ontologies to identify and resolve gaps in the Knowledge Domain, ensuring a comprehensive and complete understanding of the subject matter.
-
-
Emphasis Calibration:
-
Balancing introductory and detailed information within the Knowledge Domain to avoid biases toward high-level concepts, providing a nuanced and well-rounded perspective.
-
-
Refactoring Knowledge Inputs:
-
Restructuring of Company Knowledge into Knowledge Objects optimized for LLM tasks, enhancing the efficiency and effectiveness of the AI model's performance.
-
Achieve Value and Shape the Success of your AI Implementations in Four Essential Areas : At DBMT, we focus on four critical dimensions of value that significantly shape the success of AI implementations: Efficiency, SME Empowerment, Accuracy, and Relevance.
-
Efficiency:
-
Time is Money: Efficiency is at the core of AI's value proposition. We enable users to calculate time saved in completing tasks with AI. For example, our clients ability to implement AI-driven automation that reduces task completion time by 30%, resulting in substantial cost savings. This emphasis on efficiency is not just about speed; it's about optimizing processes to achieve more in less time.
-
-
SME Empowerment:
-
Maximizing Expertise: AI empowers SMEs by incorporating self-service capabilities for users, enabling them to handle routine tasks independently. This shift reduces the dependency on SMEs for basic inquiries, allowing them to focus on more complex and significant matters. For instance, by implementing a self-service solution, SMEs witnessed a 40% reduction in time spent answering routine queries, enabling them to channel their expertise towards strategic decision-making. The emphasis here is on utilizing SMEs more wisely, ensuring their unique skills are directed towards more high-impact, revenue generating initiatives.
-
-
Accuracy:
-
Impact of Quality Inputs: The foundation of AI accuracy lies in the quality of inputs. At DBMT, our commitment to accuracy starts with the meticulous curation of a Knowledge Domain through our unique KnowS process. KnowS ensures data inputs are consistent, complete/gap-free, structured, and generate relevant outputs. A well-structured Knowledge Domain enhances AI outputs, reducing the risk of errors and providing a solid foundation for reliable results.
-
Data-Driven Decision Confidence: AI-driven decisions are only as valuable as the accuracy of the information on which they are based. Our approach extends beyond mere reduction of errors; it instills confidence in the data-driven decisions made by your organization. Whether it's analyzing complex datasets, generating reports, or offering insights, the accuracy of our AI solutions enhance the reliability of your decision-making processes.
-
Eliminating Hallucinations: In the ever-evolving landscape of AI, hallucinations—outputs that deviate from reality—can pose significant information output risks. Through the KnowS process, we systematically identify and fill gaps in the Knowledge Domain, ensuring a complete and accurate understanding of the data. This meticulous curation significantly reduces the risk of hallucinations, providing a solid foundation for reliable AI outcomes.
-
-
Relevance:
-
Impact of Quality Inputs: Relevance in AI is not a one-size-fits-all concept; it's about tailoring outputs to the unique needs of users. Our KnowS process, by curating a Knowledge Domain that reflects the intricacies of your business, ensures AI outputs are highly relevant to users. This focus on quality inputs—structured, complete, and relevant—translates into AI-generated content that aligns precisely with user expectations.
-
User-Centric Content Generation: Relevance in AI extends beyond correctness; it delves into the user experience. Whether it's providing insights, answering queries, or generating creative content, our AI solutions are designed to be user-centric. This focus on relevance enhances user satisfaction and engagement, unlocking the full potential of AI to deliver content that aligns precisely with user expectations.
-
Dynamic Adaptation to User Prompts: AI should not just respond accurately but also adapt dynamically to evolving user prompts. Our approach to relevance involves continuous learning and refinement, ensuring that the AI model stays attuned to changes in user queries and market dynamics. This dynamic adaptation guarantees that AI-generated content remains not only accurate but consistently relevant over time.
-
DBMT’s Optimized Set of AI Tools and Solutions: DBMT offers solutions to optimize AI value, focusing on real, measurable outcomes. We provide a curated set of models, workflows, platforms and interfaces that help you stand up AI/LLM capabilities within your organization, ensuring you see a substantial return on your AI investment. DBMT tools and solutions include:
-
KnowS: DBMT’s Consulting Methodology ensures clients gain desired business value through AI/LLM.
-
AIValuePortal: DBMT’s tech stack and LLM solution leverage multiple AI/LLM models, optimized RAG, and streamlined processes.
-
AIQM: DBMT’s set of KPIs measure the quality of AI/LLM solutions based on relevance, completeness, and density of response. In AIQM, we specifically measure Accuracy and Relevance in an actionable way.
​
KnowS: DBMT’s Consulting Methodology Before diving into the specifics of KnowS, it's essential to understand the foundational approach that sets the stage for our AI/LLM solutions. KnowS is not just a methodology; it's a pathway to unlocking the full potential of AI within your organization.
-
Define Specific Use Cases:
-
Identify critical business needs that can be addressed through AI and LLMs. DBMT evaluates the complexity, potential costs, and governance requirements associated with each use case. This phase involves close collaboration with key stakeholders to pinpoint areas where AI can deliver maximum impact.
-
-
Align Stakeholders:
-
Ensure cohesive understanding and alignment among stakeholders within Business Units/Teams, clients, and technology resources. Facilitate discussions to clarify objectives, expectations, and the approach for AI implementations. Effective alignment is foundational for successful AI adoption across the organization.
-
-
Prepare Requirements:
-
Document detailed requirements for successful AI implementation. Define target users, specify output requirements, and establish governance structures. This phase ensures a clear roadmap for the AI solution, laying the groundwork for a seamless integration that aligns with organizational objectives.
-
-
Curate Input Data:
-
Identify and gather relevant documents and resources necessary to drive the AI solution. Form a foundational knowledge domain by curating data from various sources, including documents, email chains, and meeting transcripts. This step is crucial for training the AI model with accurate and comprehensive information.
-
-
Train the LLM:
-
Utilize the curated Knowledge Domain to enhance the LLM's capabilities. DBMT’s fine-tuning aligns the model with specific use cases, while Retrieval Augmented Generation (RAG) improves the model's ability to generate relevant and accurate responses. Considered a crucial phase, this step ensures that the AI model is refined and tailored to meet specific business needs.
-
-
Test the Solution:
-
Evaluate the success of the AI solution through a carefully selected subset of end-users. DBMT uses predefined metrics and criteria to assess the solution's effectiveness in addressing the identified use cases. Testing ensures that the implemented AI/LLM solution not only meets expectations but surpasses them, delivering the desired value to the organization.
-
-
Active Measurement:
-
Implement active measurement using DBMT’s AIQM measures, specifically Accuracy and Relevance. We monitor these metrics in real-time to gauge the performance of the AI solution. Additionally, we actively measure usage to govern and guide AI implementation. Usage metrics help in restricting improper use and identifying patterns of user queries, enabling continuous improvement through adjustments and additions to training and onboarding processes.
-